Efficient Microstructure Characterization of Metals using Light Microscopy: Your Questions Answered
Discover how machine learning tools can be used to overcome the challenges of characterizing new materials with multiple phases in the metallography field
17 Oct 2018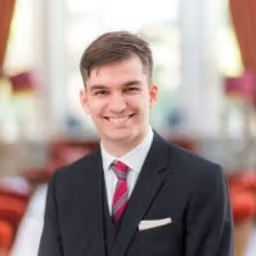
A material’s properties are strongly linked to its microstructure, such as grain size, porosity, phase and non-metallic inclusions. Light microscopy is a powerful tool for evaluating a material’s microstructure, but extracting meaningful results using traditional image analysis can be challenging, especially for new materials or materials with multiple phases. For instance, magnetic materials being developed for use in electric motors consist of complex structures. Segmentation of these structures in different phases can prove difficult with traditional image analysis techniques.
In a recent SelectScience® webinar, Tim Schubert, materials scientists at the Materials Research Institute Aalen (IMFAA), Aalen University, and Torben Wulff, solutions manager light microscopy at ZEISS Microscopy, introduce a new comprehensive solution for microstructure analysis and present standardized techniques for metallography investigation.
You can watch the webinar on demand by registering here. Read on to discover Q&A highlights from the live event
Watch Webinar NowSS: Is it well known how sectioning a material may affect a microstructure?
TS: It is very important how you section your sample for your microstructure analysis. This has two sides. The first is, if you want, for example, to measure your grain size, the international standard says you must do a cross section. If you have a rolled sheet metal, for example, you don't have to look at the elongated grains, but at the cross section of each grain to measure its size. So here the microstructure depends on the direction you do your sectioning.
The second thing is, if you cut your sample without sufficient cooling, for example, you may have a very high heat influence from the cutting and you don't get rid of this by just grinding and polishing. If you have a quenched and tempered steel, and you do dry cutting, then there is the possibility that you will get a new formed martensite, which is a completely different microstructure, and you may be led to false results on your analysis. So it is of great importance how to section your sample.
SS: Is it possible to automate the whole microscopy workflow?
TW: Yes, it's possible to automate a lot of steps in the workflow. It depends on the microscope that you use. If you have a microscope with motorized axes, motorized focus, and motorized objective turret, you can automate almost all steps, from positioning the sample under the microscope to getting the results.
SS: What steps do you need to take when using machine learning for segmentation?
TS: First of all, you have to do your preparation very well and reproducibly because your model is trained on one sort of image, one sort of etching. This is of great importance. Then you must train your model, which is normally done by an expert who knows the microstructure. With this trained model, you can then call in the material’s modules instead of threshold segmentation. You then have to set up your standard image analysis workflow or image analysis job, and that's it. Then you feed your input images, do the segmentation with the trained model, and do your standard workflow and reporting or just save your results.
SS: Can you only use the machine learning approach with images taken by light microscopes?
TW: No. The machine learning function can be called data agnostic, it doesn't depend on the type of microscope. You can use a light microscope. You can use images from electron microscopes, images from X-ray microscopes. The algorithms in the software are pixel-based and look for different features in every pixel. That is something you can use for nearly every kind of image that comes from any image source.
SS: Is it possible to know the crystal structure of composite joints to determine its mechanical properties?
TS: This is a difficult question because, normally, the crystal structure is a lot smaller than what we can image with a light microscope. For this we need to use X-ray diffraction or electron backscatter diffraction (EBSD) to find out the crystal structure. This would be applied to an SEM. With a light microscope, it is not possible or it's hard to distinguish even between face-centered cubic or body-centered cubic steels, for example, if you don't have any recrystallization twins.
SS: Why does etchant concentration matter when etching more than one sample with the same etchant? Does every etch use fresh etchant and how does your product differentiate the shape of graphite fine type A from type B?
TS: With regard to the etchant, if you use the same etchant in an etching jar for multiple samples, you vary the concentration. You get metal ions dissolved in the etchant and you take up the acid or the reactive components in your etchant. You cannot put your sample in and say, ‘If I put it in for 10 seconds at the end of the day, it will come out the same as at the beginning of the day when I used fresh etchant.’ There will be a difference, which the machine learning algorithms cannot yet cope with.
I think that there is a possibility that machine learning will be able to cope with the difference in etchant in time. But right now, we use fresh etchant and we are developing an etching cell where we have a flow of etchant so that you don't reuse your used etchant for the next etch.
TW: To answer the second part of the question, to distinguish between different graphite shapes, you look at the form and the size of every graphite particle. There are some algorithms that detect certain characteristics, like the roundness or the feret diameter, that helps you to classify the graphite into different classes.
Find out more on this topic by watching the full webinar on demand>>
SelectScience runs 3-4 webinars a month across various scientific topics, discover more of our upcoming webinars>>